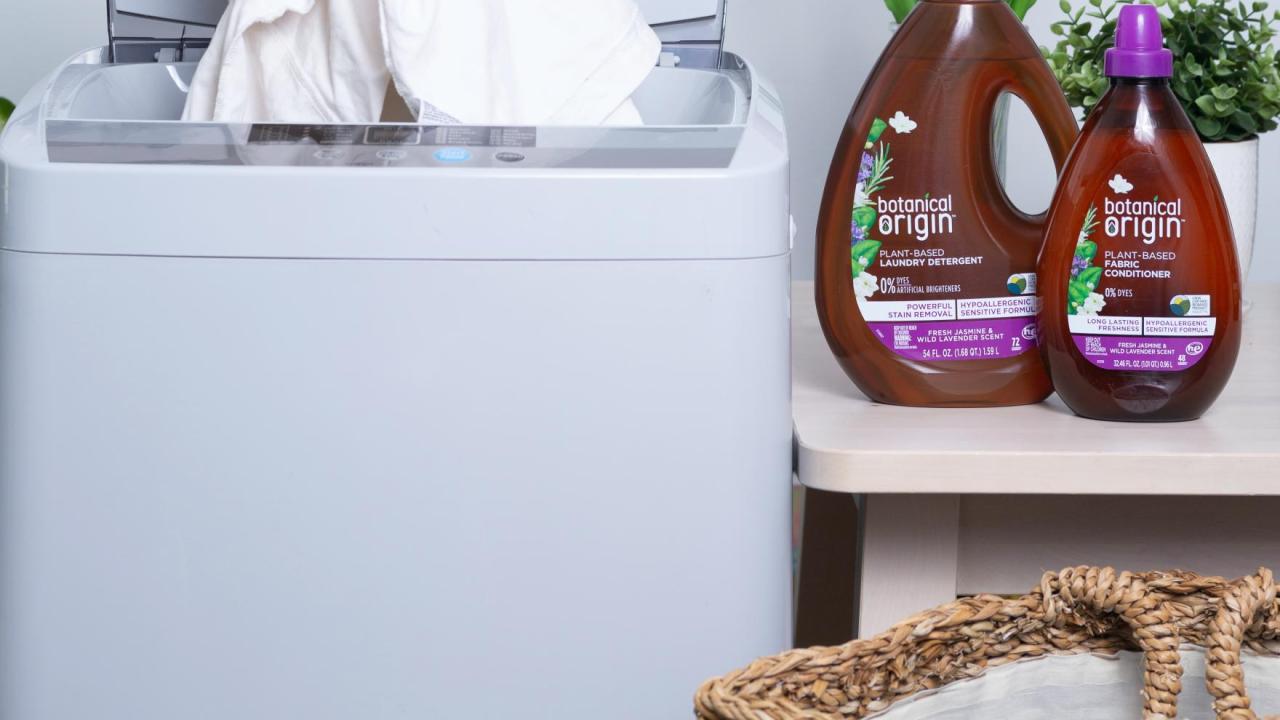
Does Your Data Spark Joy?
Understanding Data Cleaning
An often overlooked step in the process of analyzing results, data cleaning is vital to ensure the accuracy of your evaluation. After all, you put a lot of time and effort into developing your instrument, training your data collectors, and gathering a representative sample. You don't want all that hard work ruined by dirty data!
What is data cleaning?
Put simply, data cleaning is the process of removing or modifying data that is incorrect, incomplete, duplicated, or not relevant. This is important so that it does not hinder the data analysis process or skew results.
In the Evaluation Lifecycle, data cleaning comes after data collection and entry and before data analysis. However, you can think of data cleaning as a preliminary analysis of the dataset— determining which data points need to be removed or changed is a form of analysis itself!
Why does data need to be cleaned?
Data collection, like every part of evaluation, is not free from the possibility of human error. Because the data we collect now will impact policies and programs in the future, we want to be sure that our data is not leading us to false conclusions.
Often, data cleaning will involve removing duplicates or irrelevant data from the dataset. For example, if you were conducting observations of tobacco retailers, and collected observation data of the same store more than once, determining which record from that specific store should be kept in the dataset, and which should be removed, would be part of your data cleaning process.
Similarly, if you were collecting public opinion data from residents of a specific zip code, and one of your data collectors administered the survey to residents not from this population, removing responses from outside your target zip code would be necessary to ensure the accuracy of your results.
If your data is qualitative, such as notes from a key informant interview or focus group, cleaning can include increasing the readability of sentences by clarifying a respondent's meaning where necessary, turning fragments into full sentences, and writing out abbreviations and acronyms as full words. You will also likely need to remove any identifying information of respondents at this stage, especially if you are planning to utilize direct quotes in your reporting.
Things to keep in mind during data cleaning
- Always keep a record of changes made to your dataset. It's also a good idea to keep a locked copy of the raw dataset (that is, one that has had no changes made to it), in case you make a mistake during data cleaning and need to restore an entry that you initially removed. Having breadcrumbs to a backup will make this much easier, and prevent potential data loss.
- Get to know your data! The more familiar you are with the data you or your team have collected, the easier it will be to spot irregularities, outliers, and invalid or inaccurate entries. If possible, don't wait until data collection is complete to start digging into your results— you may end up having to return to the field to account for missing or invalid data.
- Be mindful of implicit bias. Data cleaning is, ultimately, a process of making decisions about your data, and changing it when needed. These decisions, and our interpretation of data, can be influenced by our own unique worldviews and cultural contexts.
TCEC is here to help!
As always, TCEC can provide guidance and technical assistance to our partners throughout the state. Email us any time at tobaccoeval@ucdavis.edu or explore our resources at https://tobaccoeval.ucdavis.edu.